We need a new Prozac: The demand for brain drug innovation
Aug. 15, 2022 · The Pharma Letter

An Expert View from Emer Leahy, chief executive, and Dani Brunner, chief innovation officer, of PsychoGenics, on the challenges involved in tackling mental disorders.
One of the greatest burdens for society, affecting millions of people, is created by unmet mental health needs. Difficult-to-treat diseases such as bipolar disorder, depression, anxiety, and schizophrenia not only affect individuals, but also their families. According to recent statistics, about one in every eight people has one or more mental or substance use disorders, with anxiety disorders topping the charts, impacting 4% of the population. Despite the enormous global burden represented by mental disorders, there remain considerable unmet needs, such as therapies for negative symptoms of schizophrenia, depression, post-traumatic stress disorder (PTSD), and more.
Funding for discovery of novel therapies, however, has not tracked the needs. For instance, the United States’ National Institute of Health budget for the Mental Health Institute, which hovers around two billion dollars, steadily decreased from 5.6% of the total budget to 4.9% from 1993 to 2020 (Figure 1). Moreover, during the last decade many large pharmaceutical companies withdrew from CNS drug discovery – although this trend has reversed in the last two to three years. While the cost of development of novel medicines is staggering, the individual and societal benefits of addressing depression and anxiety consequences are at least double that of development costs.
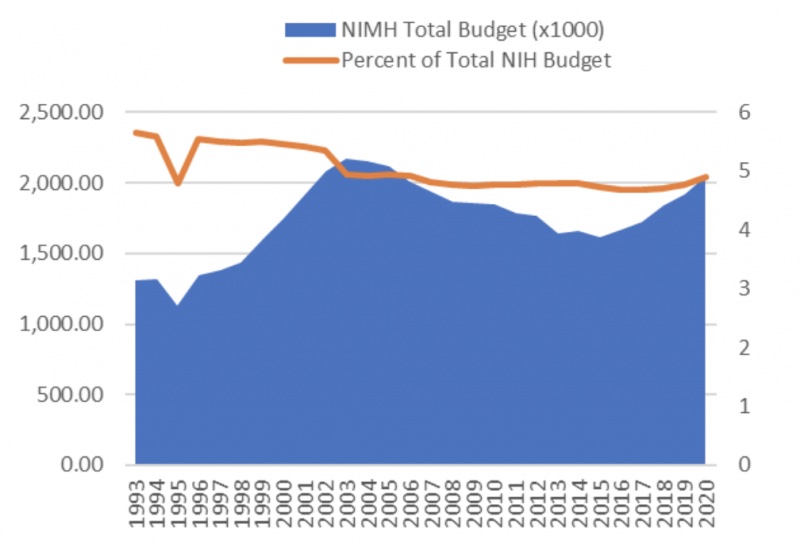
Figure 1. Budget of the United States’ National Institute for Mental Health.
Understanding complexity
Pharmaceutical companies invested in genomics as part of their drug discovery strategy, as the association of genetic risk with a particular drug mechanism increases the chances of success and approval. Genetic factors for mental disorders, whereas collectively account for a sizable proportion of disease heritability, do not reach a strong association with disease presentation on an individual basis. Moreover, mental disorders’ presentation depends on developmental, environmental, and epigenetic factors that govern disease progression. The complex etiology of CNS disorders, therefore, necessarily leads to polypharmacology. Whereas in areas such oncology, polypharmacology could be obtained through careful multitarget drug design, or through polypharmacy, the information necessary for such an approach is missing for CNS disorders.
Phenotypic drug discovery (PDD)
Looking at the new drugs approved by the US Food and Drug Administration (FDA) between 1999 and 2008, Swinney and Anthony (2011) found that the contribution of phenotypic screening for new drugs surpassed that of target-based screening, despite this era being target-based. Moreover, they argued, the main measure in target-based discovery, affinity, could not explain the multitude mechanisms represented in the approved drugs, from agonism to antagonism or allosteric modulation, from reversible to irreversible, and from competitive to non-competitive, and all combinations. They concluded that a target-based drug discovery approach for first-in-class drugs was the culprit of the high attrition rates and low productivity at the time.
The only way to truthfully capture a comprehensive profile of the effects of a candidate drug at a main target, or multiple targets, and all downstream pleiotropic consequences of such action is to explore drug activity in vivo. An in vivo PDD (iPDD) approach is an effective way to explore structure efficacy relationships of compounds with complex action in a target-agnostic manner.
PDD for CNS drug discovery
PDD allows screening whole libraries comprised of thousands of compounds with unknown target(s) to identify the starting points for drug development projects through structure efficacy relationship. PDD comprises in vitro screens using cellular assays and in vivo screens using simple organisms such as worms, flies, and zebrafish, or tests using complex organisms (rats or mice). In vivo phenotypic drug discovery platforms (iPDD) enable screening thousands of compounds to define their complex behavioral/physiological profile in a target agnostic manner. A comparison against CNS marketed drugs’ profiles then can yield their putative therapeutic potential. Targets, however, are not forgotten. For example, concurrently to efficacy screening for in an antipsychotic project using an iPDD platform, in vitro screens can be used to avoid compounds (robustly) acting at D2, 5HT1A, or 5HT2B targets, ensuring novelty and safety.
Phenotypic drug discovery for CNS
iPDD platforms such as SmartCube that collect a myriad cellular, biological, physiological, or behavioral responses under standardized processes produce large amounts of data and are amenable to machine learning approaches. For instance, data from hundreds of reference compounds, can be used to train machine learning algorithms using class labels (antidepressant, antipsychotic, toxic, etc), subclass labels (SSRI, 5HT1A agonist, etc), or drug labels (fluoxetine, risperidone, buspirone, etc) which can then provide a predictive profile to a novel compound (antidepressant, similar to an SSRI, similar to fluoxetine). iPDD thus establishes not only the potential therapeutic efficacy but also, by necessity, acceptable pharmacodynamics properties (bioavailability, minimal plasma stability and blood-brain barrier penetration).
Machine learning for CNS drug discovery
The availability of powerful computers and accessibility to large datasets have enabled a machine learning led revolution with application to target identification, target druggability prediction, compound design, compound synthesis plan design, and other areas. Machine learning can be used to design novel libraries of compounds based on medicinal chemistry insights, or novel generative models.
Privileged Scaffolds
The chemical universe of small drug-like molecules for CNS therapeutic indications is very vast, unknown, and hard to model. The combination of smart design of chemical libraries with the use of an iPDD platform allows the identification of hot zones around pharmacophores that can deliver hit rates above 20%, based on PsychoGenics experience. This is particularly high considering that virtual screening of chemical databases yields in vitro success rates rarely exceeding 1%−5%. Early high hit rate increases the success of a drug discovery project and delivers development candidates in fewer than 300 compounds tested – at a tenth of the cost when compared to target-based drug discovery (TDD).
Early analysis of all known drugs in the CMC database showed that, although the +5,000 compounds represented +1,000 different scaffolds, only 3% of the scaffolds accounted for 50% of all the drugs. From this small set of privileged scaffolds, perhaps the best known is the benzodiazepine scaffold which accounts for 15 drugs from CNS to oncology. iPDD provides medicinal chemistry insights regarding which structural features renders an “CNS active” scaffold. As most CNS scaffolds interact with multiple targets, and small modifications alters affinity at those targets and their ratios, divergent therapeutic opportunities appear (figure 2), that can only be effectively monitored with an iPDD approach.

Figure 2. Example of an early discovery phenotypic pipeline comprising three major phases. In the first phase, smart libraries of compounds are designed or identified through medicinal chemistry expertise and computational chemistry and screened with an iPDD platform. In the second phase, the results obtained from the screening of novel and marketed compounds is combed using machine learning techniques to assign therapeutic potential to the screened compounds. In the last phase, chosen compounds are tested in second tier tests to confirm therapeutic potential.
Conclusions
We argue here that iPDD platforms are necessary for CNS drug discovery. The use of machine learning for drug design, behavioral screening, and data mining enables identification of privileged structures and makes possible the delivery of developmental candidates for multiple therapeutic indications significantly faster and cheaper than a TDD approach.
Article originally published at https://www.thepharmaletter.com/article/we-need-a-new-prozac-the-demand-for-brain-drug-innovation